Table of Contents
A Brief Overview of Ice Recrystallization Inhibitors (IRIs):
Ice recrystallization inhibitors (IRIs) are a group of chemicals that play a critical role in regulating ice crystal development and expansion. IRIs’ widespread use in cryopreservation, where preserving cell viability during freezing is crucial, stems from their ability to inhibit ice crystal formation. Without interfacial reduction inhibitors (IRIs), ice crystals have the potential to expand, causing harm to cells, tissues, and other bodily substances.
Scientists’ long-term goal has been to understand the mechanisms of action of IRIs and identify more powerful ones, particularly in areas such as biotechnology, medicines, and food preservation. Datasets and data-driven approaches.
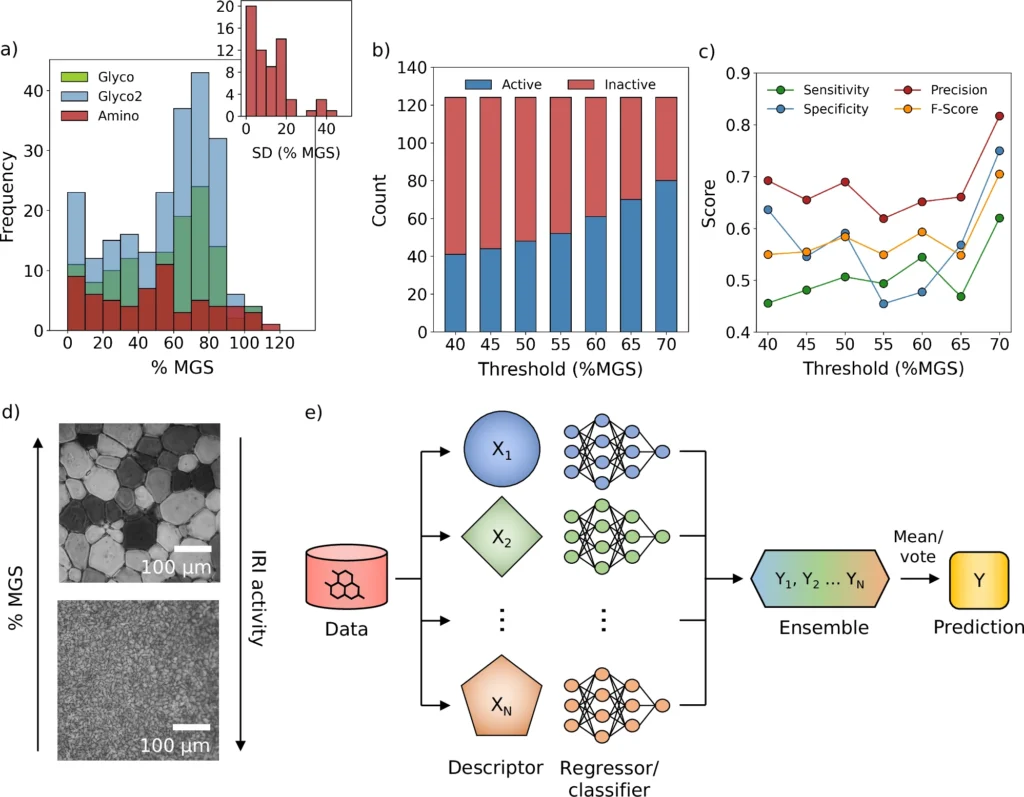
There are challenges in the process of ice recrystallization:
Iterative ice recrystallization is the process by which little ice crystals combine and grow into larger ones. This phenomenon can have particularly detrimental effects on systems that are very susceptible to the cycles of freezing and thawing, such as biological tissues or produce. During cryopreservation, the formation of massive ice crystals can puncture cell membranes, resulting in cell death.
By suppressing ice recrystallization, it is possible to save these delicate materials against erosion. Nevertheless, the task of identifying efficient inhibitors that may function in diverse situations, ranging from medical facilities to industrial uses, has been a formidable issue that has spurred thorough investigation.
An Investigation into the Function of Small Molecules in Inhibiting Ice Recrystallization:
Small compounds have identified potent possibilities for ice recrystallization inhibition. However, what exactly are they? Small molecules are organic compounds characterized by their low molecular weight, usually below 900 Daltons. These entities possess the capacity to engage with biological systems, frequently in the form of pharmaceuticals or other therapeutic substances. Within the realm of IRIs, tiny compounds are appealing due to their inherent stability, simplicity of synthesis, and adjustable functionalities.
Their compact dimensions enable accurate physical contact with ice surfaces, therefore enhancing their effectiveness in inhibiting crystal formation. Unlike macromolecules or polymers, which can also impede ice recrystallization, tiny molecules provide the advantage of scalability and reduced manufacturing expenses. Performance of cross-validated ensemble models.
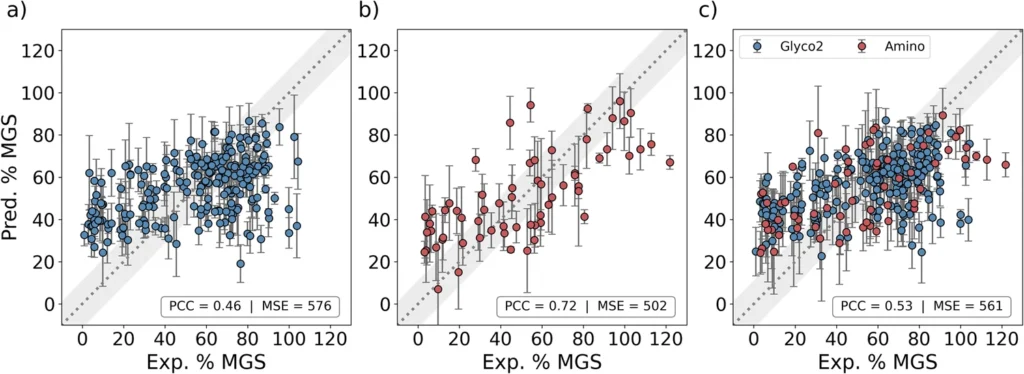
Data-driven methodologies for molecular discovery:
In the past, finding small-molecule inhibitory receptor inhibitors (IRIs) was mostly done by trial and error, which meant a lot of hard work in screening experiments to find possible candidates. However, as data-driven methodologies gain prominence, the field of molecular discovery is undergoing substantial and swift transformation.
Scientists can now predict and make new small molecules that might go through interfacial reaction interaction (IRI) more efficiently than ever before thanks to large datasets and advanced computational methods.
In drug and molecular discovery, machine learning and artificial intelligence are used:
Advanced machine learning (ML) and artificial intelligence (AI) are transforming the process of identifying tiny compounds. These methodologies may scrutinize extensive quantities of data and identify patterns that would be insurmountable for people to perceive. For instance, machine learning algorithms can analyze a large number of molecule structures to determine which ones are most likely to hinder Ice Recrystallization Inhibitors.
Artificial intelligence (AI), specifically deep learning models, exhibits exceptional efficacy in these tasks. These models help researchers find new candidates that might not have been obvious using traditional methods because they learn complex links between chemical structures and their IRI activity. Hydration property heatmap.
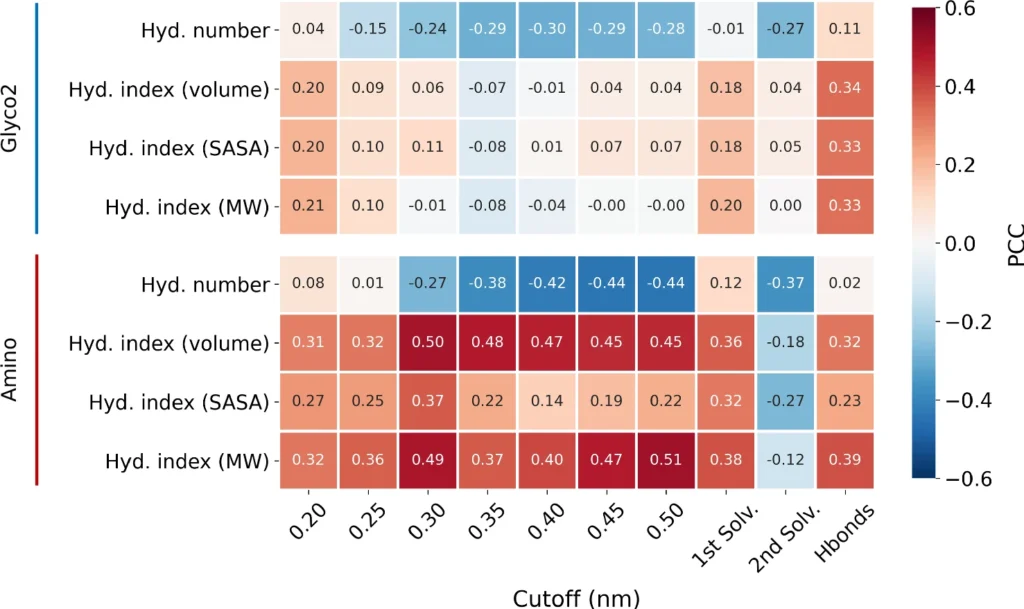
How Data-Driven Discovery Facilitates IRI Identification:
Data-driven discovery approaches depend on extensive datasets covering well-known compounds and their characteristics. By examining these records, machine learning models can detect patterns and forecast which novel small compounds may function as efficient inhibitory receptor inhibitors (IRIs).
This strategy offers the following benefits:
Computing speed: The computational screening of thousands of molecules is far faster than experimental approaches.
Accuracy: Algorithm enhancement has resulted in a consistent improvement in forecast accuracy.
The scalability of large datasets and powerful models allows for the investigation of vast chemical spaces, revealing novel opportunities.
Significant achievements in the field of data-driven small molecule discovery:
In recent years, there have been several noteworthy instances where data-driven approaches have effectively detected powerful small-molecule inhibitors of inherited resistance genes (Ice Recrystallization Inhibitors). Researchers have employed high-throughput virtual screening methods to identify new inhibitors for cryopreservation applications, resulting in improved preservation outcomes.
We are screening small molecules using high-throughput methods:
High-throughput screening (HTS) is a fundamental aspect of innovative pharmaceutical and chemical discovery. The technology enables scientists to efficiently evaluate dozens or even millions of tiny compounds against particular targets. We can employ high-temperature solidification (HTS) in combination with computer predictions to validate the effectiveness of putative candidates for ice recrystallization inhibitors.
Utilizing data-driven methodologies, HTS provides a robust tool for predicting and empirically verifying potent IRIs.
Dependent Variables That Influence the Efficacy of Small Molecule Ice Recrystallization Inhibitors (IRIs):
The effectiveness of a small-molecule immunologic response inhibitor (IRI) is dependent on several parameters, which include:
Molecular Structure: Specific structural sequences improve a molecule’s affinity for binding to ice surfaces.
Chemical Composition: A molecule’s chemical composition can influence its interaction with ice through mechanisms such as hydrophobicity, hydrogen bonding, and other chemical features.
Environmental Conditions: Environmental factors such as temperature, pH, and the existence of other solutes can alter the reactivity of an IRI molecule.
Quantitative Methods for Forecasting IRI Potency:
There are now several computational approaches accessible for predicting the potency of small-molecule inhibitory receptor inhibitors (IRIs). Advancements in molecular docking and dynamics simulations enable atomic-level interactions between molecules and ice surfaces to be predicted. Utilizing extensive datasets, machine learning models can enhance these predictions by assimilating knowledge from previous data. Ensemble predictions for novel ice recrystallization inhibitors.
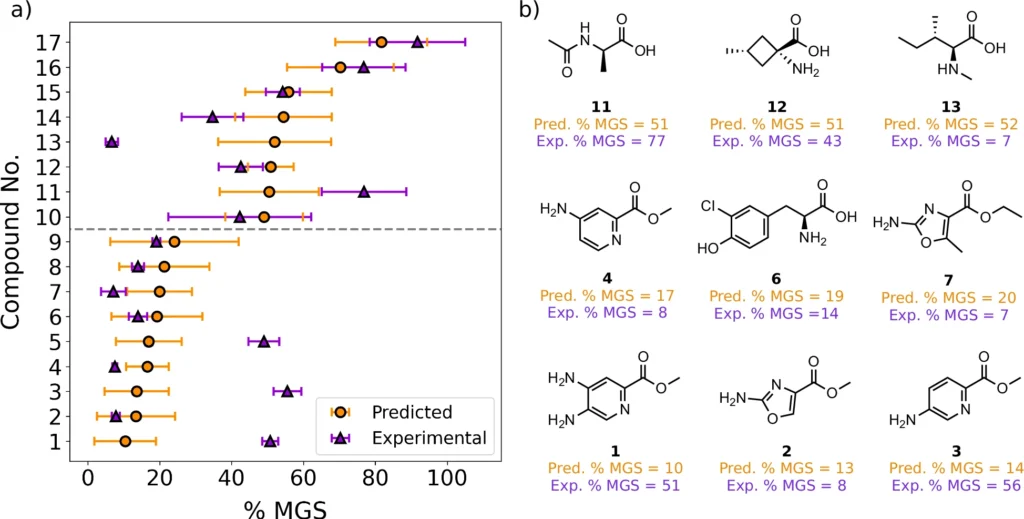
Validation of Potential IRIs via Experimental Approaches:
After identifying a possible IRI using computational techniques, it is necessary to experimentally verify its validity. We employ cryomicroscopy and differential scanning calorimetry to evaluate a molecule’s efficacy in preventing ice recrystallization under actual environmental conditions.
Data-driven Discovery of IRIs: A Critical Analysis of Challenges
Despite the great potential of data-driven discovery, there are still some obstacles to overcome:
Data Quality: Numerous databases exhibit restricted or spurious data, therefore potentially compromising the precision of forecasts.
Complexity of Ice Recrystallization: The intricate mechanisms by which molecules impede ice recrystallization remain poorly understood.
Scalability: While data-driven approaches can scale, the laboratory process of manufacturing and testing predicted compounds can still be time-consuming and costly.
Exploration of Future Directions in IRI Research:
We expect quantum computing and next-generation AI algorithms to greatly accelerate the discovery of tiny molecules, thereby enhancing the prospects of IRI research. Quantum computing has the potential to enable scientists to address molecular simulations that at present exceed the computational capabilities of classical computers.
Practical Implementations of Active Small Molecule Ice Recrystallization Inhibitors (IRIs):
Small-molecule IRIs have a wide range of potential uses, including:
Biomedical Application: The cryopreservation of cells, tissues, and even physiological organs.
Objective: To improve the longevity of frozen food products by inhibiting ice crystal development.
Climate Engineering: The prevention of ice development in critical infrastructure, such as roadways or power lines.
Summary:
The identification of tiny-molecule ice recrystallization inhibitors through data-driven analysis is a groundbreaking advancement in our capacity to safeguard delicate materials against ice-induced harm. Researchers are finding new and powerful Industrial Reaction Inhibitors (Ice Recrystallization Inhibitors) that could be useful in many areas by combining computer predictions with experimental confirmation. The ongoing development of AI and machine learning presents a promising future for this discipline.
Frequently Asked Questions:
1). What is the primary function of ice recrystallization inhibitors?
Ice recrystallization inhibitors stop the formation of ice crystals, therefore safeguarding delicate substances such as cells and tissues from freezing stress.
2). By what mechanism do tiny molecules impede Ice Recrystallization Inhibitors?
The detachment of tiny molecules from ice surfaces interferes with the fusion of small ice crystals into larger ones.
3). What are the benefits of employing a data-driven methodology in this study?
Through the analysis of extensive datasets, data-driven approaches facilitate the rapid identification and more precise forecasting of possible IRIs.
4). Are there any challenges in discovering potent small molecule IRIs?
Indeed, the obstacles encompass issues such as the quality of data, the intricacy of ice recrystallization processes, and the costly nature of experimental verification.
5). What are the anticipated future trends in IRI research?
Potential future developments include the use of quantum computing and more sophisticated artificial intelligence algorithms to improve IRI identification and optimization.
For more chemistry blogs, visit chemistry Master